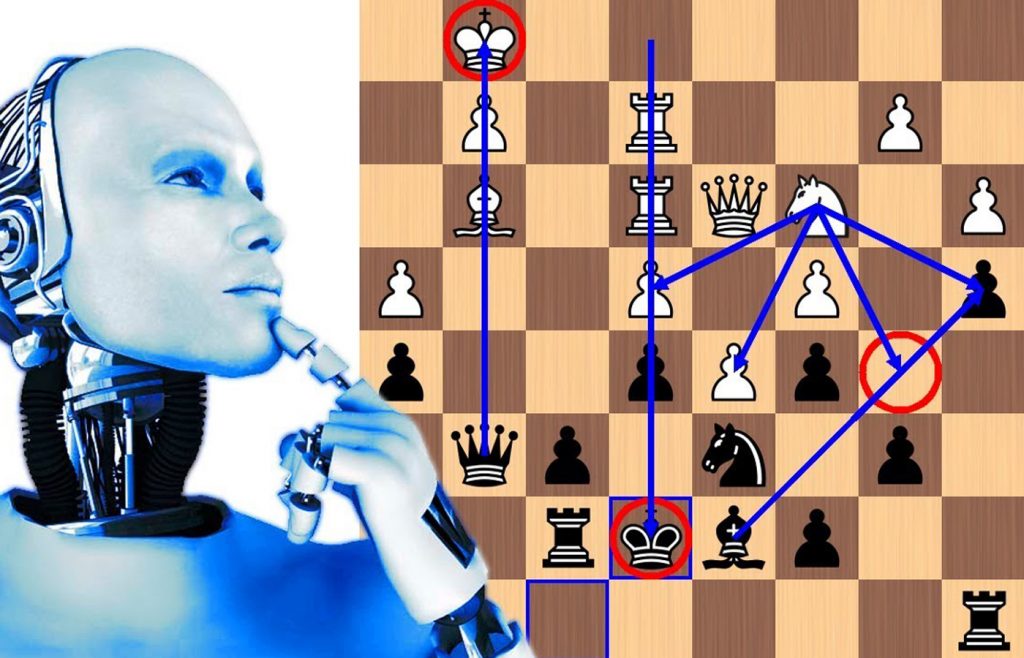
It must have been humiliating for world chess champion Gary Kasparov to lose to a computer in a six-game match back in 1997.
IBM software engineers had spent countless hours over a period of years programming Deep Blue to play master-level chess, teaching it the various openings and defenses, strategies, and nuances of the game. Deep Blue could analyze 200 million chess positions a second, a big advantage when anticipating the implications of the next possible move.
Many thought a computer could never match a human in chess—until Kasparov’s demise.
Deep Blue was eventually eclipsed, though, and Stockfish became the reigning world champion, able to defeat any and all computers and humans. Like Deep Blue, it was programmed by software engineers with a million details of chess, such as pawn structure and the importance of controlling the center of the board.
Then Google entered the picture. Using a new approach to artificial intelligence called “deep learning,” Google’s company DeepMind created AlphaZero. Instead of programming in all the nuances of the game, Google’s team programmed AlphaZero with the basic rules. Then it used a technique called “machine learning” to have the computer teach itself to become a chess master.
Using an approach to artificial intelligence called neural nets, the engineers had AlphaZero apply the simple rules of chess and play millions of games with itself, learning from its mistakes and discovering principles and effective strategies. After a total of nine hours of machine learning, AlphaZero crushed Stockfish in 100 games in 2017, winning 28 and drawing 72.
The details of the games astonished chess grandmasters. AlphaZero seemed to have gained insights that no chess master had ever conceived.
What are they? No one knows. That’s because while deep learning has been shown to be extraordinarily effective in coming up with solutions, there’s no way for the computer to share these insights.
Why stop with chess? AlphaZero became the undisputed master of Japanese chess after two hours of machine learning and master of the Chinese game of Go after 30 hours.
In recent years the application of artificial intelligence via deep learning has exploded. The ability of Google’s intelligent assistant to effectively understand what you say is based on deep learning, as is Google’s language translation service. Go to images.google.com and search on “cats,” and you’ll get a zillion photos of cats, thanks to deep learning’s ability to recognize specific images.
But some people are worried, notably the late physicist Stephen Hawking and entrepreneur Elon Musk. That’s because a computer doesn’t automatically know to avoid things that we take for granted, such as: do not kill humans.
You use deep learning to teach a computer to win at chess, and it will mindlessly pursue that goal. Here’s the scenario that scares some really smart people:
The computer, in its relentless quest to defeat man and machine at chess, harnesses whatever resources it takes to achieve that goal. Suppose, in this era of networked computers, it somehow finds its way into the computers that support the electrical grid and decides it needs those computing resources to better achieve its machine learning.
The electrical grid goes down, and people die. People in the know worry about this, especially since there are already small examples of these artificial intelligence systems doing unexpected things to achieve their goal.
For example, a system used deep learning to play the Atari game Montezuma’s Revenge. But instead of learning strategies, as with the chess example above, it found a bug in the software that allowed it to get a higher score by exploiting that bug. In another instance, an artificial intelligence system learning a game found that it could get points by falsely inputting its name as owning some of the game’s high-value items.
In short, artificial intelligence systems pursue the intended goal by sometimes acting in ways that software engineers don’t expect.
According to an excellent article in Vox, artificial intelligence systems “will work to preserve themselves, accumulate more resources, and become more efficient. They already do that, but it takes the form of weird glitches in games. As they grow more sophisticated, scientists like [computer science professor Steve] Omohundro predict more adversarial behavior.”
Of course, software engineers are increasingly anticipating these issues and trying to incorporate controls. For example, they want to figure out how to ensure that the system, in adhering to its goal, doesn’t resist being turned off. And they’re trying to program in ethical values so that the system knows not to harm life.
But it’s complicated, as you can see. I hope they get it figured out—sooner rather than later.
See column archives at JimKarpen.com.